“Some people refer to peptides, small pieces of proteins found in our cells, as the ID cards of the cell,” said Anja Conev. The Rice University Computer Science Ph.D. student and Kavraki Lab researcher is the lead author of a paper that describes a novel method for predicting how peptides bind to critical molecules involved in the development of customized immunotherapies for cancer and other diseases.
“Deep within the cell, the peptides bind to Human Leukocyte Antigen molecules. These HLA molecules are incredibly diverse, so the peptides bind to them in a variety of ways. Then the HLAs transport the peptides to the cell membrane where they broadcast the cell’s identity or contents. When cells of the immune system (T cells) scan the ID card (peptide), they immediately know if that cell is functioning as usual or if it contains something dangerous, like a virus. If a peptide-HLA molecule (pHLA) indicates danger, the contaminated cell is eliminated by the T cells,” said Conev.
Mauricio Menegatti Rigo, a Kavraki Lab postdoctoral research associate and one of the co-authors of the paper, said if the HLA does not broadcast the cell’s hazardous peptide contents, an important part of the body’s immune system response is not triggered. The variety of reasons why a threat goes undetected prompted scientists to learn more about how the peptides actually bind to HLA molecules.
“Ideally, researchers will learn to predict peptide HLA binding and thus stop threats earlier in the process,” said Rigo. “But, what if the pHLA research could also improve treatment for the patients who are already suffering?”
Wet lab scientists can already identify a patient’s unique HLA repertoire and customize immunotherapies. One example of such immunotherapy is a personalized cancer ‘peptide vaccine’ - developed to match patient’s specific HLA repertoire - which increases the likelihood of peptide-HLA binding leading to an immune response.
“One of the complexities immunologists face is that each person has a very diverse set of HLA molecules that transport these peptides,” said Conev. “This diversity is part of our immune system’s evolution; if we all had the same HLAs, we would all be susceptible to different diseases.”
“The sheer number of diverse HLAs means it is costly - in terms of time and resources - to identify the best customized drug targets for patients. Wet labs need a faster way to narrow down the target peptides that will be most beneficial to each patient’s personalized immunotherapy.”
Rigo was pleased to be part of a team of biologists, mathematicians, and computer scientists working together to solve the speedier peptide binding prediction problem.
“Our work is very relevant for the field of immunology research,” he said. “It can save time and money and enable scientists to more quickly produce a tailored treatment. This kind of result is why the interdisciplinary nature of bioinformatics appeals to me.”
Other interdisciplinary research teams have tackled the peptide binding prediction problem from different directions. Most process the streams of molecule and peptide data by interpreting the data in flat sequences for computers to access and analyze with machine learning (ML) tools. But the Rice team decided to pursue a structural method, in order to analyze the three-dimensional shapes of the actual cell components.
Conev said, “Proteins in our cells exist in 3D shapes. That is how they operate and move around. Representing them to a computer as a sequence so the computers can more easily work with the data is a simplification of what is actually happening. In our lab, we develop methods that are applied to the 3D structures of proteins and in this case, we explored pHLAs.”
She said that researchers who use the sequence-based method examine small sections of amino acids in a string, similar to breaking the data into words with nine letters where each letter represents an amino acid.
“Their analysis predicts how likely this peptide —this particular combination of letters— will bind with the HLA. Thanks to the abundance of available sequence data, these methods have been widely explored and used but they have inherent limitations,” said Conev.
“Researchers that pursue analysis of 3D or structural data have far fewer data sources - experimental derivation of this data is still expensive and slow. On top of that, each 3D structure contains a lot of information - instead of simplifying amino acids into letters, with structure we have the information of the position of every atom. Each atom is not equally important for what we are trying to predict; many of the atoms just end up adding noise and making the predictions more difficult. The most challenging part of our research is developing ML methods that will deal with this type of data and produce meaningful results for unseen pHLA structures.”
When researchers can access a lot of data to train a system, they frequently choose a ML model like neural networks. Rigo and Conev knew there was a relatively low amount of 3D pHLA data, so the Rice team would have to find or develop different ML tools.
Rigo said, “With the simpler ML models, those that can learn from less data, you have to be more thoughtful about the features you do feed into the model. We could not simply send in the 3D model data ‘as is,’ and that forced us to identify the most important aspects of the 3D model for this type of analysis. And that is where our biggest contribution lies: identifying the most critical parts of the pHLA to better predict peptide binding.”
In a video about the research, Conev explained their contributions further. She said, “We call the unique binding features ‘per peptide position’ or 3pHLA. Dividing the 3D structure into regions — groups of peptide amino acids —we characterize the regions by their energies, then feed this into our ML model. The ML models learn much more from this 3pHLA data than from any other data we’ve tried.”
Their breakthrough — identifying the importance of the per peptide position aspect of pHLA binding and developing a new method for evaluating pHLA binding, which they call 3pHLA— was particularly satisfying for Rigo. His career is directed by his desire to help scientists find more and better information in their streams of experimental lab data.
He said, “The 3pHLA breakthrough is like shining a light into a dark cave. Each person has a different set of HLAs, and this set differs from person to person. Scientists are faced with thousands of possible peptides as they attempt to customize an immunotherapy treatment plan for a patient. Now, with 3pHLA, they can both narrow the scope and shorten the time required to develop that personalized treatment.”
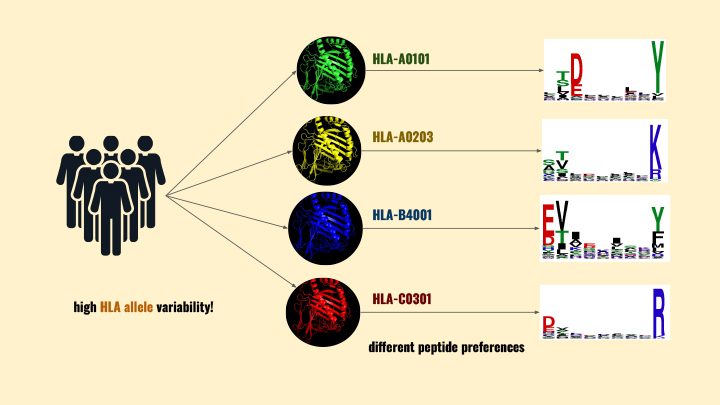
Lydia Kavraki is elated with the team’s 3pHLA research.
She said, “This work is the result of several years of research on understanding the structural aspects of protein-ligand interactions and developing computational methods for protein-ligand docking. During docking, we fit a small flexible ligand molecule – which is often a peptide in our recent work – to a protein receptor. The 3pHLA work provides an accurate way to measure how good our docking results are for receptors who play a major role for the development of personalized immunotherapy treatments.”
Rice alumnus Dinler Amaral Antunes, now an assistant professor of computational biology at the University of Houston, is co-corresponding author of the paper. Co-authors also include Didier Devaurs, again an alumnus of the Kavraki lab, who is currently a research fellow at the University of Edinburgh. Kavraki is the Noah Harding Professor of Computer Science and a professor of bioengineering, mechanical engineering and electrical and computer engineering, and director of the Ken Kennedy Institute.
Anja Conev is a Computer Science Ph.D. candidate, advised by Lydia Kavraki. She matriculated at Rice University in 2019, after completing her B.S. in Electrical Engineering from Belgrade University in Serbia.
Didier Devaurs was a postdoctoral associate at the Kavraki lab before his current position as an XDF Fellow at the University of Edinburgh. He obtained his Ph.D. in Toulouse France.
Mauricio Menegatti Rigo completed his Ph.D. in Brazil and came to Rice University in 2020 for an appointment as a Postdoctoral Research Associate. He is currently a fellow in the Computational Cancer Biology Training Program.
Dinler Amaral Antunes is an assistant professor at the Department of Biology and Biochemistry at the University of Houston. He obtained his Ph.D. in Brazil and was a postdoctoral fellow at the Kavraki Lab and a fellow of the Computational Cancer Biology Training Program.