Paris was calling and Rice University Ph.D. student Paola Cascante-Bonilla answered, flying to France in early October to present her team’s latest computer vision and language (VL) research at the 2023 International Conference on Computer Vision (ICCV 2023).
Their paper, “Going Beyond Nouns with Vision & Language Models using Synthetic Data,” introduced Synthetic Visual Concepts (SyViC), a million-scale codebase that allows its users to add synthetically generated objects like furniture and humans to an image.
“What is really exciting about SyViC is how we show — using synthetic data only — that we can train a model to perform better on complex tasks that require compositionality (how unique parts determine the composition of an object),” said Cascante-Bonilla. “A really strong aspect of SyViC is the incorporation of synthetic humans that move freely in the environment and, to some extent, can interact with the objects we place in the scene.
“This is particularly important since we saw significant boosts in the model performance only after including these digital humans — which makes sense, as most real-life pictures contain humans. In addition, we provide a fine tuning strategy for effectively leveraging SyViC toward achieving these improvements, and we perform a wide variety of ablation studies to unveil what is working, why it works, and how we made it work!”
Identifying limitations in large-scale visual language models
Cascante-Bonilla‘s enthusiasm is best understood by other computer scientists who work deep inside the world of large-scale VL models, where computer algorithms translate visual images into language — words that describe the image — and vice versa. Most of the large-scale pre-trained VL models perform remarkably well, progressing to the point where they now support zero-shot open vocabulary reasoning with natural language prompts. However, researchers have recently discovered limitations to these models due to the heavy dependence on nouns in their initial training.
“The models have difficulty understanding Visual Language Concepts (VLC) that go beyond nouns — such as attributes like ‘gray’ or actions like ‘jump.’ Another VLC challenge is compositional reasoning such as interpreting the importance of the order of words in a sentence; for example, ‘the cat is on the mat’ is not equal to ‘the mat is on the cat.’ We wanted to find a way to help VL models overcome these shortcomings without compromising their zero-shot capabilities,” said Cascante-Bonilla.
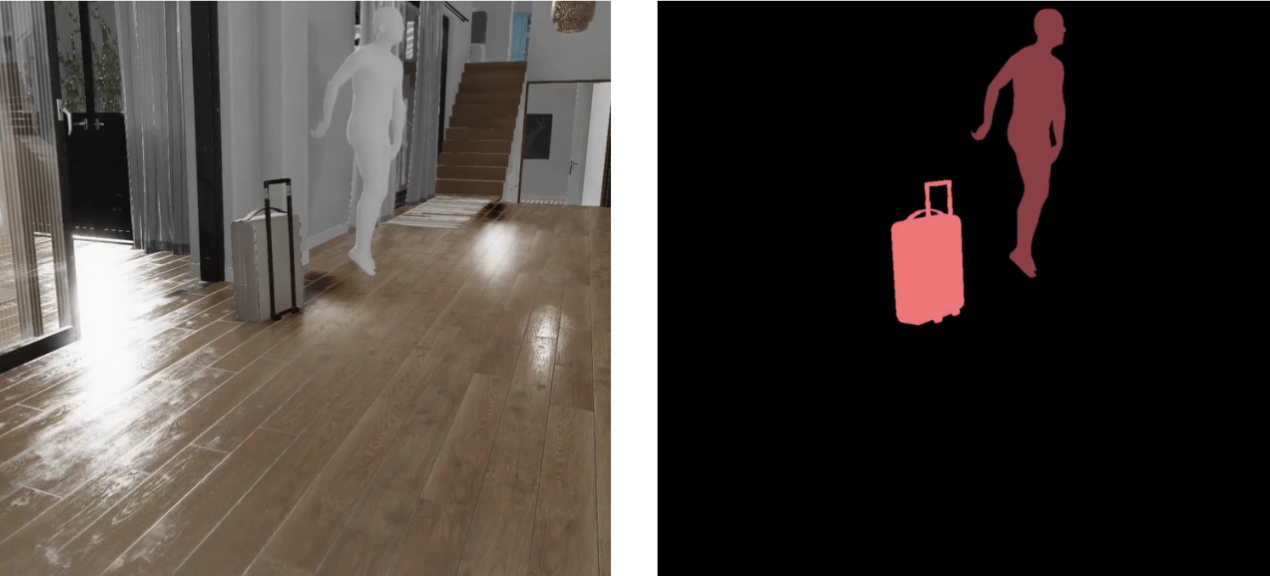
Zero-shot learning is the capacity of a model to generalize and recognize things that it has never seen before. Cascante-Bonilla said one of the models they used in their research, CLIP is an OpenAI neural network trained with 400 million image-text pairs. Because it has already ‘seen’ a lot of images with textual descriptions, the model is able to match the new text content with an image that may contain what is described.
“So, even if the model was not explicitly trained to distinguish between a cat and a dog, it contains enough information to classify each of those classes correctly. This is what we mean by zero-shot learning and this property is something we want to preserve when working with these models,” Cascante-Bonilla said.
“On the other hand, CLIP has been trained using Contrastive Learning — a training objective to ensure similar things are pulled closer together and different things are pushed away. For example, cats and tigers might get closer together but farther away from elephants and birds. While the overall results are phenomenal, prior research has shown that these models treat sentences as ‘bag-of-words’ and do not really understand the real meaning of a sentence.
“And here comes the compositionality problem, when just changing the word order of a sentence might change the meaning of it (i.e., the cat and mat combination). This order-meaning understanding comes natural for humans, but the VLC models struggle with it — even though they are very good at identifying patterns and they are matching and pushing away nouns present in text and images.”
Introducing synthetic images with auto-annotated data
Cascante-Bonilla and her colleagues believed they could improve the VLC model understanding by incorporating more annotated images, but manual annotations (in which a human describes the contents of an image) would require too much time and money. Synthetic images were the answer.
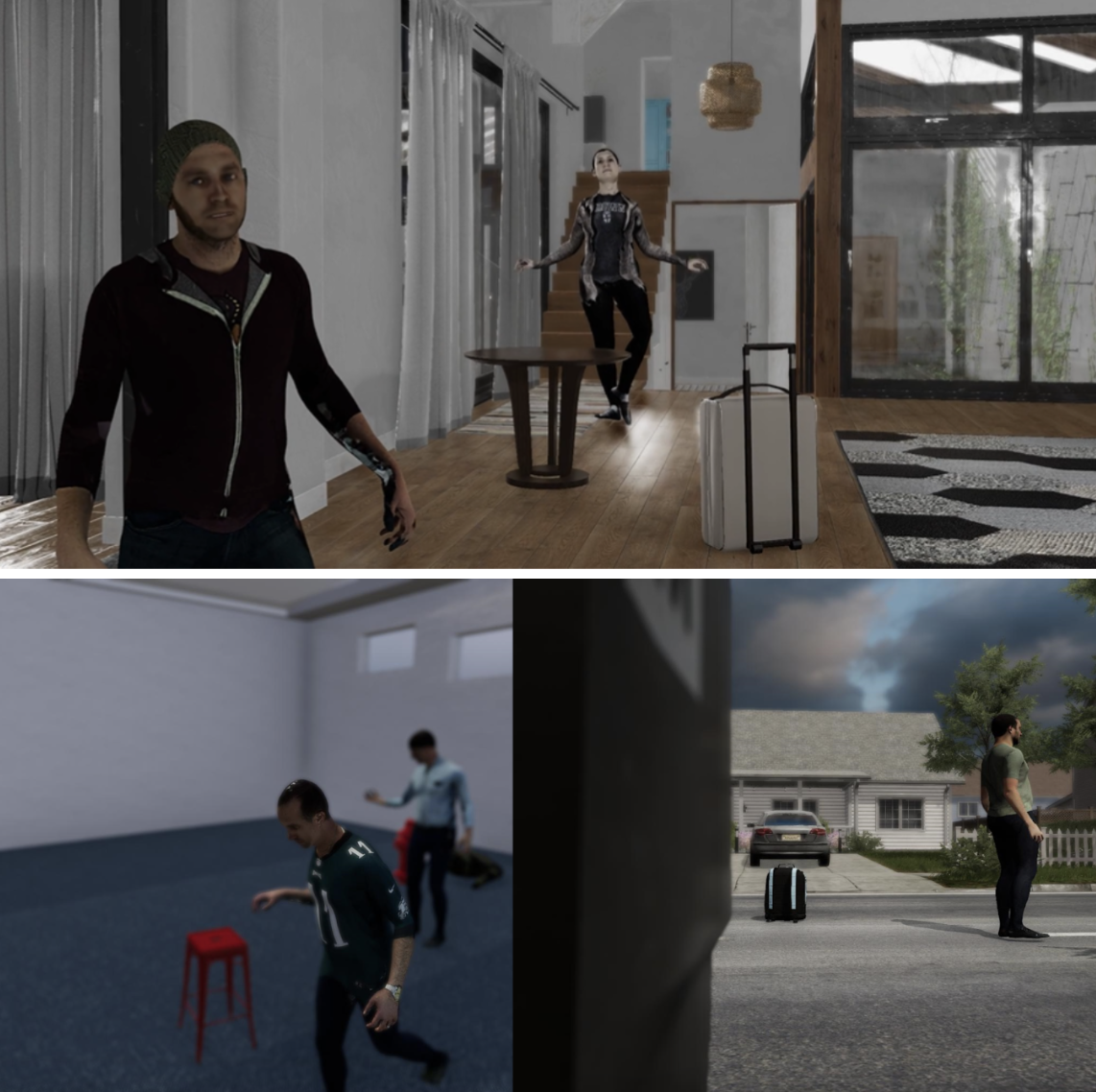
“Having access to image-text pairs is crucial for training the VL models, and one key advantage is to have clean annotated data,” she said. “Providing a pipeline to generate dense descriptions of the image data we were generating gave us a significant advantage in teaching these models about relations, actions, attributes and states of the objects in our images.
“Working with synthetic images has been a principal part of my current research; it allows for full control of your environment and you don’t have to worry about manual annotation. Our synthetic image data source comes automatically labeled due to the full control we have. You know where you are placing a chair, the material and color of the chair, and if there is something sitting on top of it. Plus, synthetic data resolves our privacy concerns.”
SyViC is the result of Cascante-Bonilla’s collaboration with her advisor, Rice CS associate professor Vicente Ordóñez, and researchers at the MIT-IMB Watson Lab, Georgia Tech, École des Ponts in Paris and the Weizmann Institute of Science in Israel.
Ordóñez said, “It was a pleasure to work with Paola and our collaborators on this project. Her leadership and work is exemplary, she has pursued an ambitious agenda that pushes the frontiers of current large scale multimodal models through clever use of synthetic and simulated data, and through novel technical methodologies.
“Paola has determination and a natural drive for success and has also had a record for mentoring other students. She was recently selected among graduating students in the area of computer vision to participate in the Doctoral Consortium at the International Conference on Computer Vision 2023 and was also selected among a large group of graduating students in all areas of electrical engineering and computer science to participate in the prestigious EECS Rising Stars 2023 Workshop.”
Cascante-Bonilla is comfortable presenting research in flagship research conferences. Last year, she introduced SimVQA at the IEEE Conference on Computer Vision and Pattern Recognition (CVPR 2022) in New Orleans. She traveled to Vancouver, Canada to present a new paper at CVPR 2023, and following her Paris ICCV presentation, she’ll return to New Orleans in December for the 2023 Conference on Neural Information Processing Systems (NeurIPS).
Her research depth and her ability to clearly explain complex computer science topics make Cascante-Bonilla a likely candidate for roles in academia, and she’s been recognized within the George R. Brown School of Engineering as a Future Faculty Fellow.
“The Future Faculty Fellows Program is a great opportunity to learn and grow. The two semesters of seminars, workshops, and mentoring activities will strengthen my academic profile and enable me to meet one-on-one with faculty across the engineering and computer science disciplines,” she said.
The EECS Rising Stars Workshop was similarly designed by MIT to identify and promote new academic talent. The annual workshop is hosted at top tier research universities around the U.S. and originally supported only 40 scholars, and although that number has almost doubled since the first 2012 event, competition for a spot in each year’s cohort remains fierce.
“Being selected to participate in the 2023 Rising Stars in EECS is an important milestone because it gathers some of the brightest EECS graduate students and postdocs around the globe,” said Cascante-Bonilla. “I look forward to all the scientific discussions, as well as sharing experiences and research ideas and nurturing future academic connections with the other participants.”